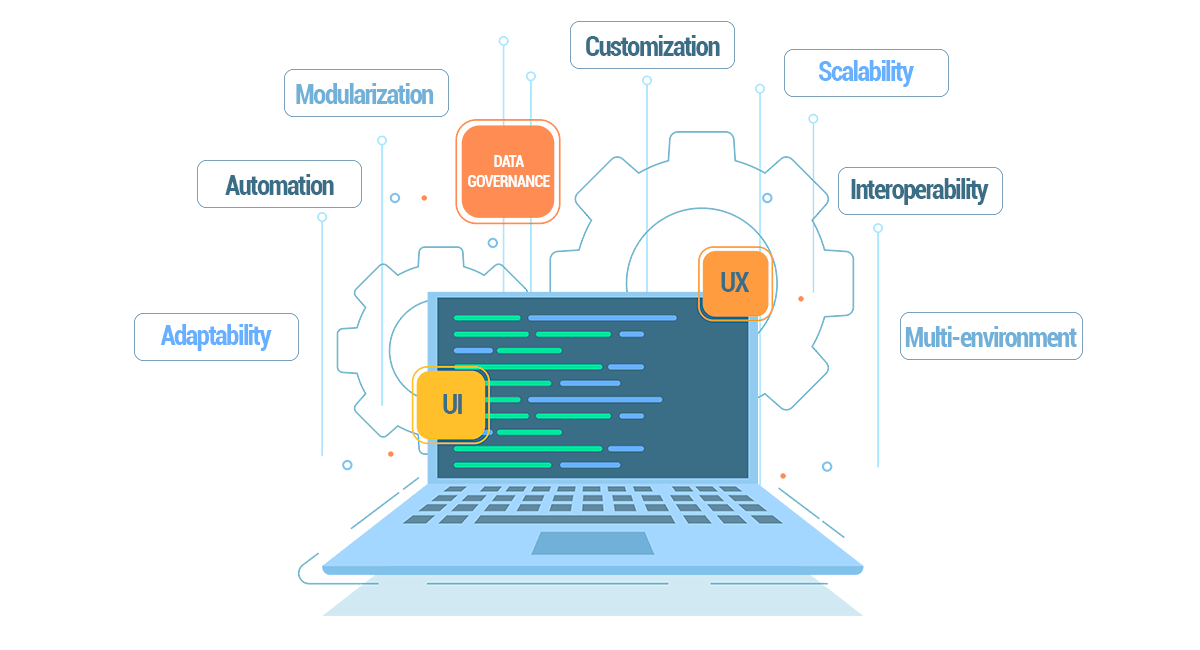
While Data Governance is fundamentally about cultural and organizational aspects and cannot be solved only by technology, technological solutions play a fundamental role and are more than necessary to achieve the implementation of an effective and efficient governance model. That is why there are many solutions on the market that work as accelerators to achieve the desired level of data governance, as well as to help organizations build and maintain that data culture necessary at all levels to reach the goal of becoming data-driven.
From version 2 of the DAMA-DMBOK we can extract some very interesting ideas:
- Organizations that establish a formal data governance program are better able to increase the value they get from their data assets
- The Data Governance function guides all other data management functions
- The purpose of Data Governance is to ensure that data is managed properly, according to policies and best practices
- Data Governance focuses on how decisions are made about data and how processes and people are expected to behave in relation to data
- Data governance is not an end in itself, it needs to aligned directly with organizational strategy
- Data governance is not a one-time thing. Governing data requires an ongoing program focused on ensuring that an organization gets value from its data and reduces risks related to data
- Data Governance is separate from IT Governance
- The goal of Data Governance is to enable the organization to manage data as an asset
- A Data Governance programme must be sustainable, embedded and measured
- Data Governance cannot be implemented overnight. It requires planning
In short, the fact that Data Governance is so linked to these cultural and organizational aspects makes it difficult and complex to evaluate the technological solutions that can support us in this area. This forces us to extend our views beyond an evaluation based on the coverage of functionalities or modules available and also consider a catalog of added value features that we should include into the assessment.
Functionalities and modules
On the one hand, if we think about functionalities and modules “designed for” Data Governance we can mention:
- Business Glossary
- Metadata management with Data Dictionary and Catalog
- Traceability and data lineage
- Architecture, design and data modeling
- Validation workflows and business process management
- Master and Reference Data Management
- Data Quality
- Data issues management
- Data security (Policies, access and use, user roles and profiling, data obfuscation)
- Dashboard with KPIs
- Management of DataLabs and Sandboxes
- Content Management and Communication Portal
- Data Services Management
- Support for Auditing
However, evaluating a solution only by the degree of completeness of these functionalities will mean that we only see part of the picture and that we can make a decision that we will regret after some time, especially when it is utopian to think that a single technological solution can accommodate all these functionalities in a self-contained way. That is why, to ensure that this does not happen, we must weigh up the functionality coverage analysis together with another type of analysis based on a set of added value features.
Added value features
These characteristics will allow us to grow in the function of Data Governance in time and form according to the specific needs of the organization:
- Automation: the processes must be as automatic as possible to reduce users manual workload and tasks.
- UX & UI: the user interface, as well as its navigation and usability should be as intuitive and friendly as possible, for all types of audiences so that any user feels comfortable using it.
- Interoperability: it must be able to share and exchange data with other systems, it must not be a “black box”, nor a hermetic component, allowing interconnection with different types of systems through connectors and allowing the use of standards.
- Customization: as configurable as possible to support the strategy and the governance model defined by the organization.
- Modularization: the different functionalities should be understood as independent pieces, so that the use of one of them does not limit the use of others, allowing the use of the necessary modules without damaging total experience.
- Multi-environment: capacity to govern multiple platforms supported by different technologies in a centralized way from the same instance.
- Scalability: adaptable as data volume increase along with the needs of processing and response, keeping performance stable over time.
- Adaptability: it must be able to adjust to the needs and the reality of the organization over time.
Additionally, from a more long-term point of view, the characteristics which we should pay special attention and concentrate on are:
- Vendor lock-in: as far as possible, we must try to ensure that the solutions selected do not “tie” the organization to a single vendor by acquiring large dependencies and thus avoid migration from one solution to another with great consequences.
- Learning curve: being powerful solutions, the learning curve should not be a problem for the users, who should not invest a great number of hours in learning the use of the solution or need very specific and expensive trainings and certifications.
- User limit: in case we want to extend data governance to the whole organization we must consider solutions that do not license by users since this can result in a limited use of the solution as the costs increase in relation to the increase of users and not based on the real use.
- Cost of licenses: the cost must be flexible and scalable, tending to be under a pay-per-use model, allowing total control over the ROI without consider a high initial investment to maximize time-to-market and time-to-value.
What can we find in the market?
Looking at the market, given that we are talking about technology, storage and data processing solutions vendors usually offer modules oriented to data governance within their own platforms, but generally with a biased vision and low interoperability, becoming a problem of integration between technologies and resulting in a new challenge for the governance of applications and technology.
On the other hand, given the existing need in the market, in recent years new vendors have emerged that specialise in the development of specific and independent solutions with an agnostic vision from data storage and processing technologies, providing this practice with a new set of tools to facilitate its execution. This group includes, for example, Anjana Data.
In spite of this, due to the complexity and extent of the practice, the solutions are usually focused on offering a set of functionalities and specific capacities and it seems very difficult, if not impossible, to find a single solution that covers everything. Therefore, it is advisable to look for the different pieces that help us build the puzzle of solutions that supports Data Governance based on the needs of the organization, starting with the most critical aspects.
In addition, the market for specific “Data Governance” solutions has not existed for long and is not very widespread, except in the USA where they do represent a high volume of business. In fact, for both Gartner and Forrester there is not yet a quadrant or a wave respectively in this area, placing the solutions between “Metadata Management”, “Master Data Management” and “Data Quality”.
Finally, within the spectrum of Data Governance solutions vendors, we can group them into different groups… but this is something that gives for another complete article 😊